Table Of Content
- What is DOE? Design of Experiments Basics for Beginners
- What does “main effects” refer to?
- Blocking and Confounding in 2k Design
- DOE Masterclass: Design of Experiments 101 for biologists.
- One-Way/Single Factor Analysis of Variance, ANOVA
- Use screening experiments to reduce cost and time
- Response surface methodology (RSM) designs
- Statistical experiments, following Charles S. Peirce
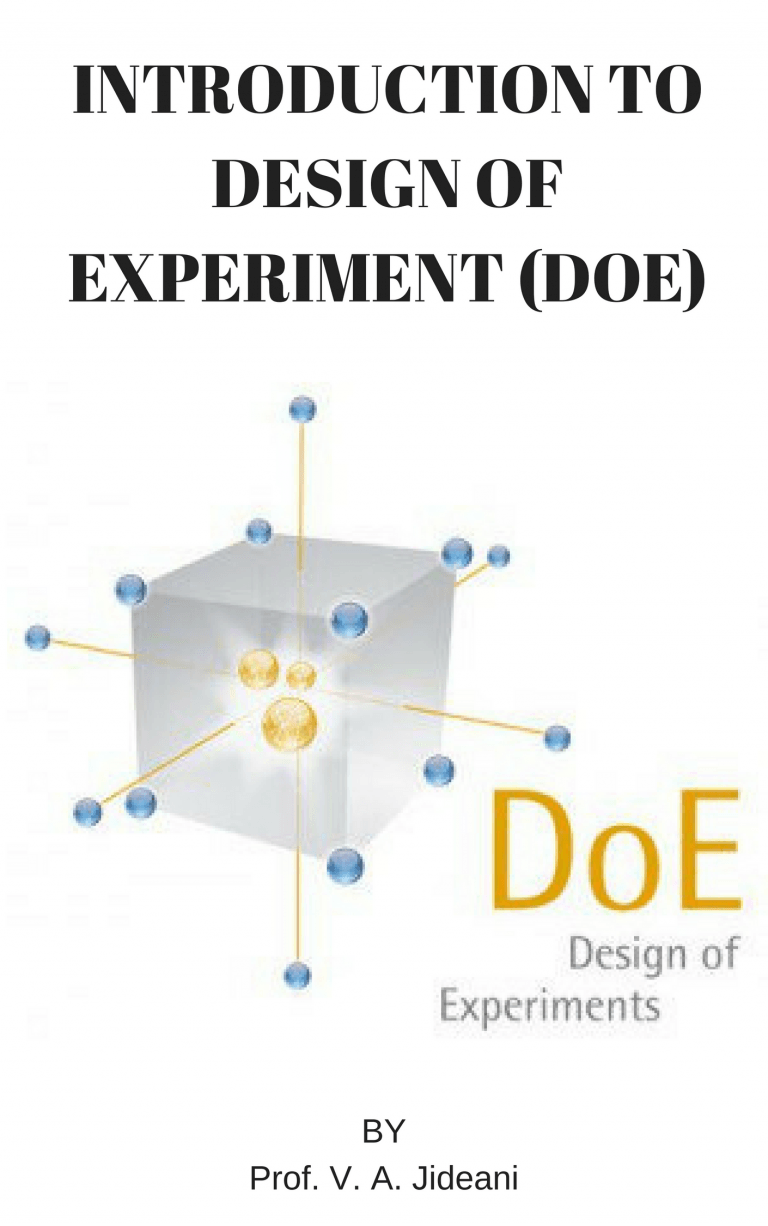
The lady was randomly given four cups in which tea was poured before the milk and four where the milk was poured first. Except where otherwise noted, content on this site is licensed under a CC BY-NC 4.0 license. The prerequisite for this course is STAT Regression Methods and STAT Analysis of Variance.
What is DOE? Design of Experiments Basics for Beginners
Because one component of DoE is the settings of factors, performing an experimental runs are applicable here. With DoE, you can determine the effects of changes made with the factors and their levels that influences the response. Excess variations in the process are the cause of added expense. With DoE, factors are identified, responses are interpreted, and waste is eliminated or changed. Conducting experimental design allows you to look at different alternatives.
What does “main effects” refer to?
Below is an example of a table that shows the yield that was obtained when changing the volume from 500 to 700 ml. In the scatterplot on the right, we have plotted the measured yield against the change in reaction volume, and it doesn’t take long to see that the best volume is located at 550 ml. This translates into a small amount of additional experimental work and can be a useful pattern when iterating from stage to stage of your DOE campaign. This number tells you what “order”—or in non “DOE speak”, the size—of interactions you can distinguish within a particular effect. In other words, whether you can tell one 2-factor from another 2-factor interaction. Fractional factorial DOE is not, however, suitable for sophisticated modeling.
Blocking and Confounding in 2k Design
Blocking involves grouping similar experimental units and randomizing treatments within these blocks. This technique increases the experiment’s precision by controlling for block variation, allowing for a more accurate assessment of the treatment effects. Blocking showcases each experiment’s meticulous design and thoughtful consideration, highlighting the beauty in organizing complex data into understandable and meaningful patterns. Randomization is assigning subjects or experimental units to different groups in a study purely by chance. This critical process ensures that each group is comparable and extraneous variables do not bias the results.
How to Design and Run a Controlled Experiment - Cannabis Business Times
How to Design and Run a Controlled Experiment.
Posted: Thu, 08 Nov 2018 15:09:40 GMT [source]
We will understand that we should reposition the experimental plan according to the dashed arrow. So the problem with the COST approach is that we can get very different implications if we choose other starting points. We perceive that the optimum was found, but the other— and perhaps more problematic thing—is that we didn’t realize that continuing to do additional experiments would produce even higher yields. Zooming out and picturing what we have done on a map, we can see that we have only been exploiting a very small part of the entire experimental space. The true relationship between pH and volume is represented by the Contour Plot pictured below. We can see that the optimal value would be somewhere at the top in the larger red area.
RSM designs allow you to build a predictive model of your system’s response surface. You can then use the predictive model to find the factor settings or region that will optimize your response. Discover the profound impact of the ‘Lady Tasting Tea’ experiment in statistics and data science, shaping modern hypothesis testing methods. Analysis of the experimental results revealed that welding temperature and pressure were the most significant factors influencing joint strength, with a notable interaction effect between them.
It acknowledges that the clarity and aesthetics of data presentation can illuminate insights, making them accessible and impactful to a broader audience. FMCG industry is a part of consumer goods industry that includes all the products which are sold to the general public by any means such as retail stores, internet or by phone. These are mostly used by the consumers in their daily life and may include food, drinks, health and hygiene, cosmetics, household appliances, among others. DoE helps in comparing alternatives or options to get the response where price will be cheaper but does not compromise on quality.
In the pharmaceutical industry, DOE is most typically used throughout the drug formulation and manufacturing phases. Qualitty is critical for drug products because health and safety of consumers are at risk when a product doesn’t meet the standards. DoE is used in drug testing, reducing impurities in the process of making drugs, before releasing it for consumer use.
Statistical experiments, following Charles S. Peirce
Instead of covering all the designs in detail, we’ll start you off by covering the most commonly used and important DOE designs (Figure 1). As your campaign progresses, the DOE design types involve investing more experimental effort to answer more detailed questions. If you’re struggling with statistics while analyzing data for your projects, this is your ultimate solution for Data Analysis! Ensure the safety of workers and the quality of your products and services with regular quality assurance training. They enlisted the company’s Master Black Belt to help them do the experiment using a two-level approach. This article will explore two of the common approaches to DOE as well as the benefits of using DOE and offer some best practices for a successful experiment.
We can see three main reasons that DOE Is a better approach to experiment design than the COST approach. In this way, DOE allows you to construct a carefully prepared set of representative experiments, in which all relevant factors are varied simultaneously. The important thing here is that when we start to evaluate the result, we will obtain very valuable information about the direction in which to move for improving the result.
The main effects of a DOE are the individual factors that have a statistically significant effect on your output. In the common two-level DOE, an effect is measured by subtracting the response value for running at the high level from the response value for running at the low level. Unfortunately, most process outcomes are a function of interactions rather than pure main effects. You will need to understand the implications of that when operating your processes. Based on this, you can fine-tune the experiment and use DOE to determine which combination of factors at specific levels gives the optimal balance of yield and taste.
You need to begin by setting a realistic range for each factor. So, testing 1kg of plant food could prove toxic and expensive. Strawberries also need plenty of water to ensure juiciness; applying 1ml of water would be difficult to accurately achieve and, possibly, trigger drought stress responses. In this course we will pretty much cover the textbook - all of the concepts and designs included. I think we will have plenty of examples to look at and experience to draw from. JMP links dynamic data visualization with powerful statistics.
In those cases, researchers must be aware of not certifying about causal attribution when their design doesn't allow for it. The same goes for studies with correlational design (Adér & Mellenbergh, 2008). Lactobacillus paracasei IMC502® is a commercially successful probiotic strain, however, there are no reports that investigate growth medium composition in relation to improved biomass production for this strain. The major outcome of the present study is the design and optimization of a growth medium based on vegan components to be used in the cultivation of Lactobacillus paracasei IMC502®, by using Design of Experiments (DoE). Besides comparing different carbon sources, the use of plant-based peptones as nitrogen sources was considered.
Throughout this exploration of the Design of Experiments (DoE), we’ve unveiled the methodology’s profound capability to refine research methods, enhancing precision in data analysis and discovering inherent truths. From ensuring unbiased data through randomization and enhancing experimental reliability via replication to the meticulous design showcased by blocking, DoE embodies a holistic approach to scientific inquiry. It rests on a philosophical foundation that values truth in measurement, goodness in methodology, and beauty in data visualization, all while upholding the highest ethical standards. This journey through DoE’s essential components, varied experimental designs, and innovative software tools, punctuated by a case study, illustrates its transformative impact across fields. There are multiple approaches for determining the set of design points (unique combinations of the settings of the independent variables) to be used in the experiment. Factorial Design explores every possible combination of factors and levels within a single experiment, providing comprehensive data on the main effects and interactions between factors.
No comments:
Post a Comment