Table Of Content
- Blocking and Confounding in 2k Design
- main types of DOE designs, explained
- DOE looks at different ranges within factors.
- Random-Effect Models, Mixed Models, Nested, Split-Plot & Repeated Measure Design of Experiments
- Implementing Design of Experiments (DOE): A practical example
- SafetyCulture (formerly iAuditor) for Experimental Design
- Book traversal links for 1.3 - Steps for Planning, Conducting and Analyzing an Experiment

Fractional factorial designs will provide limited interaction information because you did not test all the possible combinations. But, what if you aren’t able to run the entire set of combinations of a full factorial? What if you have monetary or time constraints, or too many variables? This is when you might choose to run a fractional factorial, also referred to as a screening DOE, which uses only a fraction of the total runs. That fraction can be one-half, one-quarter, one-eighth, and so forth depending on the number of factors or variables.
Blocking and Confounding in 2k Design
DoE is a useful tool for determining specific factors affecting defect levels in a product, which may be used to improve the design of the product. With DoE, the factors and their levels are checked and see which of them when used are giving the exact quality in the response. When discussing the proper settings for your process variables, people often rely on what they have always done, on what Old Joe taught them years ago, or even where they feel the best setting should be.
main types of DOE designs, explained
The Japanese car industry adopted statistical quality control procedures and conducted experiments which started this new era. Total Quality Management (TQM), Continuous Quality Improvement (CQI) are management techniques that have come out of this statistical quality revolution - statistical quality control and design of experiments. A design of experiments (DOE) is a set of statistical tools for planning, executing, analyzing, and interpreting experimental tests to determine the impact of your process factors on the outcomes of your process.
DOE looks at different ranges within factors.
Regardless of who or what is involved in the process - it is still going to work. We will come back to this notion of robustness later in the course (Lesson 12). All experiments are designed experiments, it is just that some are poorly designed and some are well-designed.
Fractional Factorial Design reduces the number of experimental runs required by selecting a subset of the complete factorial design. This approach is optimal for initial exploratory studies where the goal is to identify the most significant factors with a limited budget or time frame. DOE statistical outputs will indicate whether your main effects and interactions are statistically significant or not. You will need to understand that so you focus on those variables that have real impact on your process.
The experimentation using all possible factor combinations is called a full factorial design. Design of experiments (DOE) is a systematic, efficient method that enables scientists and engineers to study the relationship between multiple input variables (aka factors) and key output variables (aka responses). It is a structured approach for collecting data and making discoveries. Many factorial designs add a single central point for each factor to help determine whether there is curvature. In DoE, experiments are being used to find an unknown outcome or effect, to test a theory, or to demonstrate an already known effect.
SafetyCulture (formerly iAuditor) for Experimental Design
MoreSteam gave a simple illustration to explain the components of the experiment—the three aspects that need to be analyzed in the design experiments—and understanding the meaning of each is crucial in defining DoE. A unique application of DOE in marketing is called conjoint analysis. A web-based company wanted to design its website to increase traffic and online sales. Doing a traditional DOE was not practical, so leadership decided to use conjoint analysis to help them design the optimal web page.
Book traversal links for 1.3 - Steps for Planning, Conducting and Analyzing an Experiment
In other words, interactions between 2 factors—are more common and more influential than higher order effects (typically, interactions between more than 3 factors). A fractional factorial design takes a rational sample of the experimental landscape to provide a balanced, structured design that generates explanatory and predictive models. The study of the design of experiments is an important topic in metascience. Statistics is often taught as though the design of the data collection and the data cleaning have already been done in advance. This course is created to provide an understanding of how experiments should be designed so that when the data are collected, these shortcomings are avoided. In the Design of Experiments (DoE), selecting the right software tools is pivotal for ensuring precision, efficiency, and aesthetic clarity of data analysis.
Identifying interactions
The importance of statistical quality control was taken to Japan in the 1950s by W Edward Deming. This started what Montgomery calls a second Industrial Era, and sometimes the quality revolution. After the second world war, Japanese products were of terrible quality.
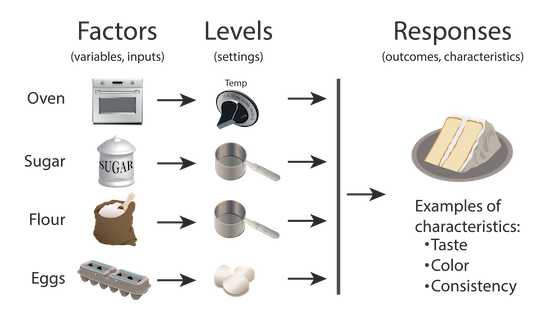
In addition to treatment factors, there are nuisance factors which are not your primary focus, but you have to deal with them. Sometimes these are called blocking factors, mainly because we will try to block on these factors to prevent them from influencing the results. What this course will deal with primarily is the choice of the design. This focus includes all the related issues about how we handle these factors in conducting our experiments. As well as these savings, DOE achieves higher precision and reduced variability when estimating the effects of each factor or interaction than using OFAT. It also systematically estimates the interaction between factors, which is not possible with OFAT experiments.
Immediately following World War II the first industrial era marked another resurgence in the use of DOE. It was at this time that Box and Wilson (1951) wrote the key paper in response surface designs thinking of the output as a response function and trying to find the optimum conditions for this function. And, an interesting fact here - he married Fisher's daughter! He worked in the chemical industry in England in his early career and then came to America and worked at the University of Wisconsin for most of his career. Implementing the Design of Experiments (DoE) comes with challenges and ethical considerations, each requiring careful attention to maintain research integrity and respect for the data and subjects involved. Addressing these aspects is crucial for the credibility of DoE outcomes and for upholding the principles of scientific research that honor truth, contribute to societal welfare, and appreciate the beauty of discovery.
Master Outlier Detection and Treatment to enhance your data analysis skills. Master the Student’s t-test to accurately compare population means, ensuring valid conclusions in your research. While the challenges of implementing DoE are non-trivial, they can be effectively managed with meticulous planning, ethical consideration, and adherence to scientific principles. Beauty in data visualization is the principle that recognizes the power of well-presented data to convey complex truths elegantly and effectively.
The use of design of experiments with multiple responses to determine optimal formulations for in vivo hepatic mRNA ... - ScienceDirect.com
The use of design of experiments with multiple responses to determine optimal formulations for in vivo hepatic mRNA ....
Posted: Tue, 10 Nov 2020 08:00:00 GMT [source]
Michael Sadowski, aka Sid, is the Director of Scientific Software at Synthace, where he leads the company’s DOE product development. In his 10 years at the company he has consulted on dozens of DOE campaigns, many of which included aspects of QbD. It’s so clear, it’s easy to distinguish pixels that are sitting next to each other. Now, imagine you're looking at the same picture with a much lower resolution. It’s grainy, and it’s impossible to identify one pixel from the next. The beauty of DOE is that by choosing a DOE design, you have also chosen the type of analysis you will do.
It helps in making an informed decision on what to use or what to change. This methodology can also be used to discover the best combination of alternatives in the experiment. Use existing data and data analysis to try and identify the most logical factors for your experiment. Regression analysis is often a good source of selecting potentially significant factors. Design of experiments allows you to test numerous factors to determine which make the largest contributions to yield and taste. Factors might include preheating the oven, baking time, ingredients, amount of moisture, baking temperature, etc.-- what else?
Another way is to reduce the size or the length of the confidence interval is to reduce the error variance - which brings us to blocking. Specify how you can manipulate the factor and hold all other conditions fixed, to insure that these extraneous conditions aren't influencing the response you plan to measure. Test different settings of two factors and see what the resulting yield is.
Goodness in methodology goes beyond the technical, embedding an ethical framework within which experiments are designed and conducted. It is a commitment to integrity, ensuring that the methods employed are both scientifically valid and morally sound, respecting the dignity of all participants and the sanctity of the natural world being studied. Unless you’ve done some prior screening of your potential factors, you might want to start your DOE with a screening or fractional factorial design.
No comments:
Post a Comment